
Transforming Software License Management through AI

Revolutionizing Software License Optimization
To address this challenge, they partnered with intive to develop a strategy and create a PoC for optimizing software license costs. Leveraging intive's expertise, the entertainment enterprise sought to reduce substantial software license costs by accurately predicting the actual license needs of thousands of employees across hundreds of applications. This required analyzing a vast dataset of over 2 million logins to licensed tools and associated metadata to understand historical usage patterns.In just 8 weeks, intive delivered a fully functional, robust PoC, leveraging expertise in data-driven solutions and advanced technology, laying the foundation for substantial global cost savings.

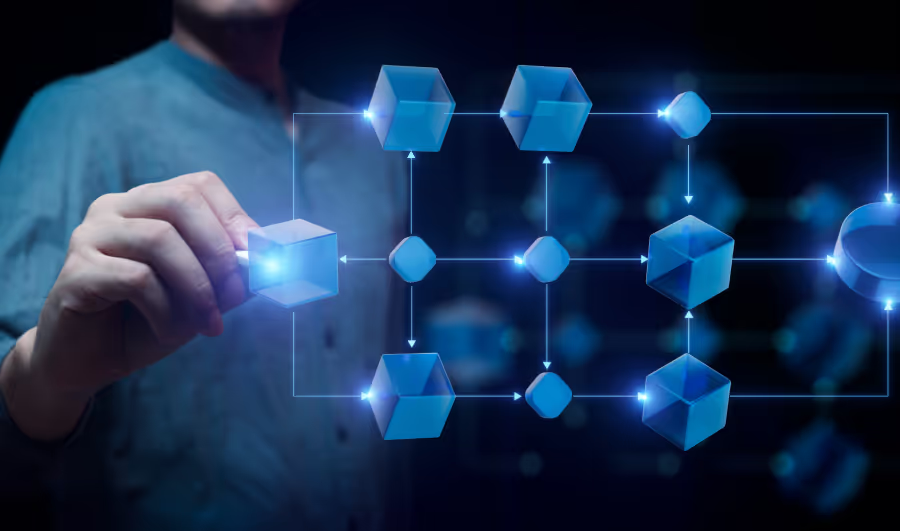
The team developed a serverless ETL pipeline, meticulously crafted to collect, format, and consolidate vast amounts of usage data logs, user profiles, and app profiles, exceeding 500GB.
intive strategically deployed application usage models on cloud infrastructure using a serverless architecture. This deployment not only facilitated real-time analysis of user profiles but also enabled precise predictions of usage likelihood, all while significantly reducing infrastructure overhead. By leveraging the benefits of serverless cloud infrastructure, intive ensured efficient processing of requests, thereby enhancing the accuracy and efficiency of usage likelihood predictions.
Drawing on expertise in machine learning (ML) and artificial intelligence (AI), intive developed decision-tree-based models tailored to each application within the ecosystem. Trained on historical usage data, these models accurately predicted the likelihood of users requiring licenses, facilitating optimal resource allocation and seamless alignment with the client's needs.
intive's models went beyond basic predictions by incorporating sophisticated algorithms and AI techniques.
These advanced capabilities enabled the models to automatically identify key data fields such as department, business unit, job title, and location. This approach enhanced the transparency and explainability of predictions, providing valuable insights to guide strategic decision-making effectively throughout the project lifecycle.
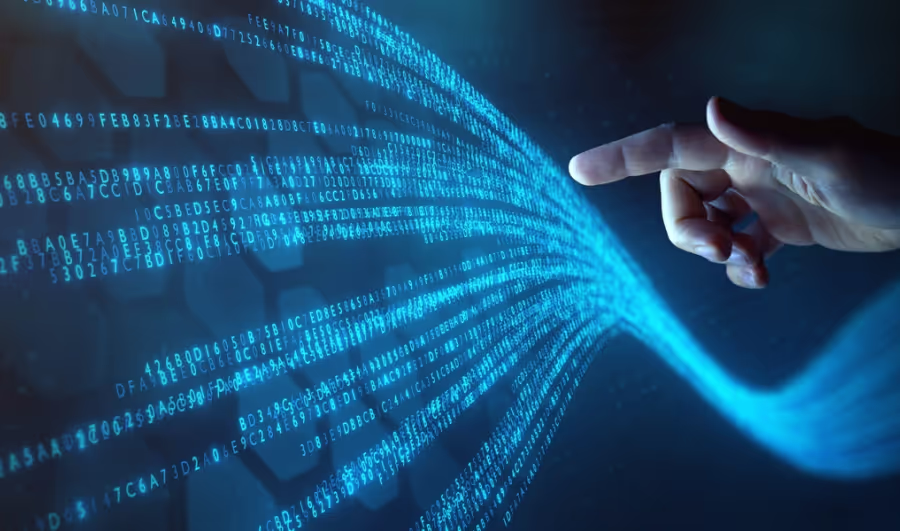

Leveraging the model-predicted likelihood of usage enabled precise allocation of software licenses to each user. This streamlined approach ensured optimal resource allocation, eliminating unnecessary expenditures on unused licenses. Furthermore, the PoC showcased the potential for significant cost savings, illustrating the financial impact of the solution. Through the analysis of licenses for a single tool, projected annual savings of over hundreds of thousands of dollars were identified, demonstrating the potential for substantial cost reductions on a wider application. This calculation represented the potential savings from optimizing just one software application, highlighting the substantial cost reductions achievable across the entire software portfolio when applying intive's predictive model.